We are a bioinformatics research lab in the Center for Computational Biology and the Department of Biomedical Engineering at Johns Hopkins University. We are also affiliated with the Department of Computer Science, the Center for Imaging Science, the Kavli Neuroscience Discovery Institute, and more.
We develop methods for analyzing spatially resolved transcriptomic sequencing and imaging data.
Spatial organization at both the subcellular-level within cells as well as the cellular-level within tissues play important roles in regulating cell identity and function. Recent technological advances have enabled high-throughput spatially resolved transcriptomic profiling at single-molecule and near-single-cell resolution. We develop machine learning and other statistical approaches as open-source computational software to take advantage of this new spatial information in deriving biological insights regarding how spatial organization plays a role in both healthy and diseased settings.
- Gohta Aihara, Kalen Clifton, Mayling Chen, Zhuoyan Li, Lyla Atta, Brendan F Miller, Rahul Satija, John W Hickey, Jean Fan^. SEraster - a rasterization preprocessing framework for scalable spatial omics data analysis. Bioinformatics. June 20, 2024. doi.org/10.1093/bioinformatics/btae412
- Lyla Atta, Kalen Clifton, Manjari Anant, Gohta Aihara, and Jean Fan^. Gene count normalization in single-cell imaging-based spatially resolved transcriptomics. Genome Biology. June 12, 2024. doi.org/10.1186/s13059-024-03303-w
- Kalen Clifton*, Manjari Anant*, Gohta Aihara, Lyla Atta, Osagie K Aimiuwu, Justus M Kebschull, Michael I Miller, Daniel Tward^, Jean Fan^. STalign - alignment of spatial transcriptomics data using diffeomorphic metric mapping. Nature Communications. December 8, 2023. doi.org/10.1038/s41467-023-43915-7
- Brendan F Miller, Feiyang Huang, Lyla Atta, Arpan Sahoo, Jean Fan^. Reference-free cell type deconvolution of pixel-resolution spatially resolved transcriptomics data. Nature Communications. 2022. doi:/10.1038/s41467-022-30033-z
- Lyla Atta, Arpan Sahoo, Jean Fan^. VeloViz: RNA-velocity informed embeddings for visualizing cellular trajectories. Bioinformatics. 2021. /doi:10.1093/bioinformatics/btab653
- Lyla Atta, Jean Fan^. Computational challenges and opportunities in spatially resolved transcriptomic data analysis. Nature Communications. 2021. doi:10.1038/s41467-021-25557-9
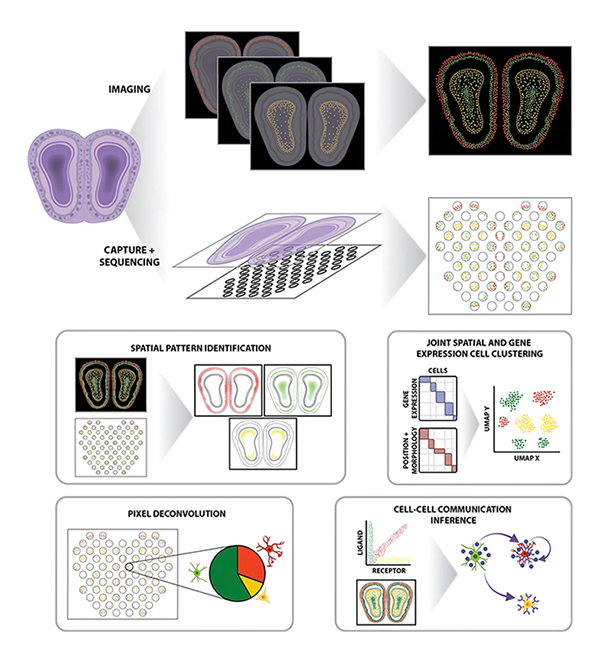
We apply these methods to better understand the impact of cellular heterogeneity on cancer pathogenesis and prognosis.
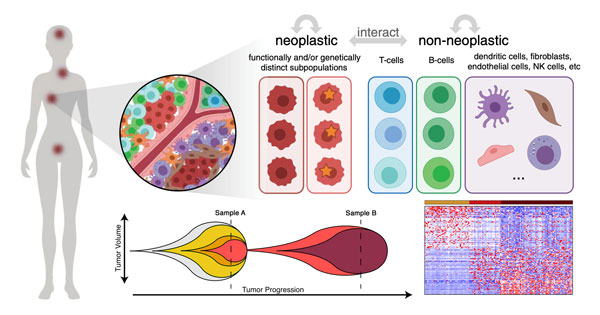
Advancements in high-throughput sequencing and imaging technologies have uncovered tremendous genetic, epigenetic, transcriptional, and spatial heterogeneity in various cancers but their impact on clinical outcomes is not well understood. We establish close collaborations with clinical collaborators to develop and apply bioinformatics methods that contribute to a more complete understanding of how cellular heterogeneity impacts tumor progression, therapeutic resistance, and ultimately clinical prognosis. We are particularly interested in pediatric gliomas.
- Jean Fan^, Kamil Slowikowski, Fan Zhang. Single-cell transcriptomics in cancer - computational challenges and opportunities. Nature Experimental and Molecular Medicine. 2020, doi.org:10.1038/s12276-020-0422-0
- Jean Fan*, Hae-Ock Lee*, Soohyun Lee, Da-eun Ryu, Semin Lee, et al. Linking transcriptional and genetic tumor heterogeneity through allele analysis of single-cell RNA-seq. Genome Research. 2018. doi:10.1101/gr.228080.117
- Lili Wang*, Jean Fan*, Joshua M. Francis, George Georghiou, Sarah Hergert, et al. Integrated single-cell genetic and transcriptional analysis suggests novel drivers of chronic lymphocytic leukemia. Genome Research. 2017. doi:10.1101/gr.217331.116
Latest Publications
- SEraster - a rasterization preprocessing framework for scalable spatial omics data analysis on 20 June 2024
- Gene count normalization in single-cell imaging-based spatially resolved transcriptomics on 12 June 2024
- Single-cell morphology encodes functional subtypes of senescence in aging human dermal fibroblasts on 16 May 2024
- STalign - alignment of spatial transcriptomics data using diffeomorphic metric mapping on 08 December 2023
- Soluble PD-L1 reprograms blood monocytes to prevent cerebral edema and facilitate recovery after ischemic stroke on 07 December 2023
Latest News
- Gohta Aihara graduates from the lab and will be heading to California to pursue his PhD at Caltech! Thanks for being a part of the team and best of luck in your future pursuits! on 28 June 2024
- Prof. Fan gives an invited talk in the Advances in Spatial Technologies session at the Single-Cell Cancer Biology and also serves on the faculty mentoring panel to discuss Shaping the Future of Single-Cell Cancer Biology. on 11 June 2024
- JEFworks lab members give talks at the 2024 HuBMAP Annual Meeting. on 14 May 2024
- JEFworks lab members celebrate the end of the semester with a lab BBQ. on 03 May 2024
- Prof. Fan gives an invited talk at STATGEN in the session on new mathematical paradigms behind single-cell biology. on 30 April 2024
Latest Blog Posts
- Characterizing spatial heterogeneity using spatial bootstrapping with SEraster on 23 July 2024
- I use R to (try to) figure out which hospital I should go to for shoppable medical services by comparing costs through analyzing Hospital Price Transparency data on 22 April 2024
- Cross modality image alignment at single cell resolution with STalign on 11 April 2024
- Spatial Transcriptomics Analysis Of Xenium Lymph Node on 24 March 2024
- Querying Google Scholar with Rvest on 18 March 2024